What is the Difference Between Artificial Intelligence & Machine Learning?
Rohan Roy
Jan 2, 2022
AI/ML






In a world with virtual assistants and smart robots, nearly every man is familiar with the terms artificial intelligence and machine learning. We know that AI & ML are the hearts behind every savvy system that stuns us, but most of us still have no idea what they are and what actually differentiates them from each other.
The terms artificial intelligence and machine learning are often used interchangeably today since people think that they’re basically the same when they aren’t. The poor definitions are what arises the confusion between AI & ML.
While artificial intelligence is a vast area of topics, machine learning is only a small part of it. AI is about building a computer system that can mimic human intelligence so that pre-programming is not required and algorithms that can work with their own intellect are used in such systems.
The tasks that come under “artificial intelligence” include problem-solving, learning, and planning which are accomplished through feeding data to the algorithms so that they can replicate such behaviors of the human brain.
With the surge in the amount of information being generated and available for analysis, researchers found that this data can be used to teach machines to perform tasks and then give them access to the internet and all the information it holds to learn themselves.
This gave birth to machine learning, which comprises the learning part of AI unlike replicating the actual human behaviors. It is a subset of AI that fosters a computer system to take decisions and make predictions based on the past data collected and just like AI systems, pre-programming isn’t required.
Before ML algorithms, AI programs were only able to perform and automate low-level tasks. With the advent of ML, AI could execute tasks beyond what they were programmed to do and the systems started evolving with each iteration.
ML algorithms can analyze large chunks of data quickly unlike the human brain, making pattern detection and prediction much easier. Structured and semi-structured data are fed into the machine learning algorithms so that they can recognize patterns in the occurrence of results and produce accurate predictions analyzing those patterns. ML algorithms change over time and get better at tasks as they interpret more and more data.
While AI covers a large set of domains and can handle multiple, complex tasks, machine learning algorithms are restricted to specific domains on which they’re trained. For instance, if we generate and train an algorithm using existing data to differentiate plants from animals based on their features, it’ll be restricted to doing that single task and will be unresponsive to other data.

Types of AI
Artificial intelligence is classified into three essential groups based on its capabilities; narrow, general, and strong AI.
Narrow AI enables the computer system to perform a specific task and cannot perform beyond its scope. Virtual assistants which operate with a limited pre-defined range of functions are an instance of narrow AI. Speech recognition, image recognition, and self-driving cars are some other examples.
General AI can perform intellectual tasks efficiently just like a human. It aims at making a system as smart as a human and currently such a system doesn’t exist which can perform tasks as perfectly as a human. General AI is still under development since researchers aren’t still able to develop a system even as intelligent as a six-year-old.
Super AI comprises systems that can beat human intelligence and can perform tasks better than any human. This can be seen as a product of general AI. The ability to think, reason, solve puzzles, learn, make decisions are all characteristics of super AI.
Use Cases of AI
Personal assistants: Assigning basic tasks and simple daily chores to virtual assistants have already become a part of our daily routine. The virtual assistant market is expanding quickly and tech giants are competing to take over the market which is going to grow in demand in the future. Even though there are concerns about user privacy, people still don’t seem to hate their Siri, Alexa, or Google Home.
Digital marketing: Though this is a new scene for AI, companies have already started deploying AI bots to optimize their marketing activities. AI tools are used for content generation in written, audio, or video format, and leaving such tasks to AI is turning out to save time and resources for companies.
Customer Service: For customers wanting instant answers to their questions, AI chatbots are proving to be very effective. Powered with voice recognition, and personalized recommendations, these chatbots are pre-programmed to answer any basic frequently asked queries and guide the customer through the website in case of an issue.
Types of ML
There are three types of machine learning, based on the nature of data available for training the algorithms; supervised, unsupervised, and reinforcement learning.
In supervised learning, the ML algorithm is trained using accurately labeled data from the training dataset. This training dataset should be similar to the dataset that we’ll input later to solve problems. The algorithm will find relationships between the parameters in the training data and deploy these findings on the final dataset.
Unsupervised learning works on raw, unlabeled data, enabling working on large datasets, without the need of them being machine-readable. Unlike supervised learning, instead of finding relationships in the training data, unsupervised learning algorithms discover the hidden structures and patterns and uncover insights from them.
Reinforcement learning is very similar to the learning process of a human. Here, the algorithm is introduced into a work environment instead of feeding already existing data. It is a feedback-based, trial and error method, where the algorithm is reinforced for every right output and discouraged for every wrong output.
Use Cases of ML
User behavior analysis: The large amount of data generated by businesses over the years can be put to good use using machine learning algorithms. Such data can be used to predict purchasing habits, market trends, and other useful insights. Later, these will be helpful in decision making, streamlining inventory management, optimizing marketing platforms, and so on.
Upgrading Automation: Machine learning algorithms can evaluate the existing manufacturing models and discover their shortcomings and pain points to improve the automation of such processes. Combining automation techniques with machine learning can lead to automation processes that are constantly improving.
Cyber Security: ML algorithms can predict the nature of future cyberattacks by studying past attack data and finding the vulnerabilities in the system. Also, incorporating ML in spam filters can improve the accuracy of the filter as the efficiency of the algorithm increases with each filtering.
Conclusion
Looking through the types and applications of AI and machine learning, we can see that they overlap a lot in terms of their working and their functions. AI & ML are not that different from each other, as ML is essentially a part of AI. It concludes that all ML is AI, but not all AI is ML.
Artificial intelligence along with machine learning can power up every industry and enterprise by automating tasks, generating valuable insights, and efficiently managing resources. Eventually, as artificial intelligence evolves, powered with ML, it’ll be able to do tasks better than humans. Soon, most of the repetitive, high-volume, and rule-based business processes will be taken over by AI.

In a world with virtual assistants and smart robots, nearly every man is familiar with the terms artificial intelligence and machine learning. We know that AI & ML are the hearts behind every savvy system that stuns us, but most of us still have no idea what they are and what actually differentiates them from each other.
The terms artificial intelligence and machine learning are often used interchangeably today since people think that they’re basically the same when they aren’t. The poor definitions are what arises the confusion between AI & ML.
While artificial intelligence is a vast area of topics, machine learning is only a small part of it. AI is about building a computer system that can mimic human intelligence so that pre-programming is not required and algorithms that can work with their own intellect are used in such systems.
The tasks that come under “artificial intelligence” include problem-solving, learning, and planning which are accomplished through feeding data to the algorithms so that they can replicate such behaviors of the human brain.
With the surge in the amount of information being generated and available for analysis, researchers found that this data can be used to teach machines to perform tasks and then give them access to the internet and all the information it holds to learn themselves.
This gave birth to machine learning, which comprises the learning part of AI unlike replicating the actual human behaviors. It is a subset of AI that fosters a computer system to take decisions and make predictions based on the past data collected and just like AI systems, pre-programming isn’t required.
Before ML algorithms, AI programs were only able to perform and automate low-level tasks. With the advent of ML, AI could execute tasks beyond what they were programmed to do and the systems started evolving with each iteration.
ML algorithms can analyze large chunks of data quickly unlike the human brain, making pattern detection and prediction much easier. Structured and semi-structured data are fed into the machine learning algorithms so that they can recognize patterns in the occurrence of results and produce accurate predictions analyzing those patterns. ML algorithms change over time and get better at tasks as they interpret more and more data.
While AI covers a large set of domains and can handle multiple, complex tasks, machine learning algorithms are restricted to specific domains on which they’re trained. For instance, if we generate and train an algorithm using existing data to differentiate plants from animals based on their features, it’ll be restricted to doing that single task and will be unresponsive to other data.

Types of AI
Artificial intelligence is classified into three essential groups based on its capabilities; narrow, general, and strong AI.
Narrow AI enables the computer system to perform a specific task and cannot perform beyond its scope. Virtual assistants which operate with a limited pre-defined range of functions are an instance of narrow AI. Speech recognition, image recognition, and self-driving cars are some other examples.
General AI can perform intellectual tasks efficiently just like a human. It aims at making a system as smart as a human and currently such a system doesn’t exist which can perform tasks as perfectly as a human. General AI is still under development since researchers aren’t still able to develop a system even as intelligent as a six-year-old.
Super AI comprises systems that can beat human intelligence and can perform tasks better than any human. This can be seen as a product of general AI. The ability to think, reason, solve puzzles, learn, make decisions are all characteristics of super AI.
Use Cases of AI
Personal assistants: Assigning basic tasks and simple daily chores to virtual assistants have already become a part of our daily routine. The virtual assistant market is expanding quickly and tech giants are competing to take over the market which is going to grow in demand in the future. Even though there are concerns about user privacy, people still don’t seem to hate their Siri, Alexa, or Google Home.
Digital marketing: Though this is a new scene for AI, companies have already started deploying AI bots to optimize their marketing activities. AI tools are used for content generation in written, audio, or video format, and leaving such tasks to AI is turning out to save time and resources for companies.
Customer Service: For customers wanting instant answers to their questions, AI chatbots are proving to be very effective. Powered with voice recognition, and personalized recommendations, these chatbots are pre-programmed to answer any basic frequently asked queries and guide the customer through the website in case of an issue.
Types of ML
There are three types of machine learning, based on the nature of data available for training the algorithms; supervised, unsupervised, and reinforcement learning.
In supervised learning, the ML algorithm is trained using accurately labeled data from the training dataset. This training dataset should be similar to the dataset that we’ll input later to solve problems. The algorithm will find relationships between the parameters in the training data and deploy these findings on the final dataset.
Unsupervised learning works on raw, unlabeled data, enabling working on large datasets, without the need of them being machine-readable. Unlike supervised learning, instead of finding relationships in the training data, unsupervised learning algorithms discover the hidden structures and patterns and uncover insights from them.
Reinforcement learning is very similar to the learning process of a human. Here, the algorithm is introduced into a work environment instead of feeding already existing data. It is a feedback-based, trial and error method, where the algorithm is reinforced for every right output and discouraged for every wrong output.
Use Cases of ML
User behavior analysis: The large amount of data generated by businesses over the years can be put to good use using machine learning algorithms. Such data can be used to predict purchasing habits, market trends, and other useful insights. Later, these will be helpful in decision making, streamlining inventory management, optimizing marketing platforms, and so on.
Upgrading Automation: Machine learning algorithms can evaluate the existing manufacturing models and discover their shortcomings and pain points to improve the automation of such processes. Combining automation techniques with machine learning can lead to automation processes that are constantly improving.
Cyber Security: ML algorithms can predict the nature of future cyberattacks by studying past attack data and finding the vulnerabilities in the system. Also, incorporating ML in spam filters can improve the accuracy of the filter as the efficiency of the algorithm increases with each filtering.
Conclusion
Looking through the types and applications of AI and machine learning, we can see that they overlap a lot in terms of their working and their functions. AI & ML are not that different from each other, as ML is essentially a part of AI. It concludes that all ML is AI, but not all AI is ML.
Artificial intelligence along with machine learning can power up every industry and enterprise by automating tasks, generating valuable insights, and efficiently managing resources. Eventually, as artificial intelligence evolves, powered with ML, it’ll be able to do tasks better than humans. Soon, most of the repetitive, high-volume, and rule-based business processes will be taken over by AI.

In a world with virtual assistants and smart robots, nearly every man is familiar with the terms artificial intelligence and machine learning. We know that AI & ML are the hearts behind every savvy system that stuns us, but most of us still have no idea what they are and what actually differentiates them from each other.
The terms artificial intelligence and machine learning are often used interchangeably today since people think that they’re basically the same when they aren’t. The poor definitions are what arises the confusion between AI & ML.
While artificial intelligence is a vast area of topics, machine learning is only a small part of it. AI is about building a computer system that can mimic human intelligence so that pre-programming is not required and algorithms that can work with their own intellect are used in such systems.
The tasks that come under “artificial intelligence” include problem-solving, learning, and planning which are accomplished through feeding data to the algorithms so that they can replicate such behaviors of the human brain.
With the surge in the amount of information being generated and available for analysis, researchers found that this data can be used to teach machines to perform tasks and then give them access to the internet and all the information it holds to learn themselves.
This gave birth to machine learning, which comprises the learning part of AI unlike replicating the actual human behaviors. It is a subset of AI that fosters a computer system to take decisions and make predictions based on the past data collected and just like AI systems, pre-programming isn’t required.
Before ML algorithms, AI programs were only able to perform and automate low-level tasks. With the advent of ML, AI could execute tasks beyond what they were programmed to do and the systems started evolving with each iteration.
ML algorithms can analyze large chunks of data quickly unlike the human brain, making pattern detection and prediction much easier. Structured and semi-structured data are fed into the machine learning algorithms so that they can recognize patterns in the occurrence of results and produce accurate predictions analyzing those patterns. ML algorithms change over time and get better at tasks as they interpret more and more data.
While AI covers a large set of domains and can handle multiple, complex tasks, machine learning algorithms are restricted to specific domains on which they’re trained. For instance, if we generate and train an algorithm using existing data to differentiate plants from animals based on their features, it’ll be restricted to doing that single task and will be unresponsive to other data.

Types of AI
Artificial intelligence is classified into three essential groups based on its capabilities; narrow, general, and strong AI.
Narrow AI enables the computer system to perform a specific task and cannot perform beyond its scope. Virtual assistants which operate with a limited pre-defined range of functions are an instance of narrow AI. Speech recognition, image recognition, and self-driving cars are some other examples.
General AI can perform intellectual tasks efficiently just like a human. It aims at making a system as smart as a human and currently such a system doesn’t exist which can perform tasks as perfectly as a human. General AI is still under development since researchers aren’t still able to develop a system even as intelligent as a six-year-old.
Super AI comprises systems that can beat human intelligence and can perform tasks better than any human. This can be seen as a product of general AI. The ability to think, reason, solve puzzles, learn, make decisions are all characteristics of super AI.
Use Cases of AI
Personal assistants: Assigning basic tasks and simple daily chores to virtual assistants have already become a part of our daily routine. The virtual assistant market is expanding quickly and tech giants are competing to take over the market which is going to grow in demand in the future. Even though there are concerns about user privacy, people still don’t seem to hate their Siri, Alexa, or Google Home.
Digital marketing: Though this is a new scene for AI, companies have already started deploying AI bots to optimize their marketing activities. AI tools are used for content generation in written, audio, or video format, and leaving such tasks to AI is turning out to save time and resources for companies.
Customer Service: For customers wanting instant answers to their questions, AI chatbots are proving to be very effective. Powered with voice recognition, and personalized recommendations, these chatbots are pre-programmed to answer any basic frequently asked queries and guide the customer through the website in case of an issue.
Types of ML
There are three types of machine learning, based on the nature of data available for training the algorithms; supervised, unsupervised, and reinforcement learning.
In supervised learning, the ML algorithm is trained using accurately labeled data from the training dataset. This training dataset should be similar to the dataset that we’ll input later to solve problems. The algorithm will find relationships between the parameters in the training data and deploy these findings on the final dataset.
Unsupervised learning works on raw, unlabeled data, enabling working on large datasets, without the need of them being machine-readable. Unlike supervised learning, instead of finding relationships in the training data, unsupervised learning algorithms discover the hidden structures and patterns and uncover insights from them.
Reinforcement learning is very similar to the learning process of a human. Here, the algorithm is introduced into a work environment instead of feeding already existing data. It is a feedback-based, trial and error method, where the algorithm is reinforced for every right output and discouraged for every wrong output.
Use Cases of ML
User behavior analysis: The large amount of data generated by businesses over the years can be put to good use using machine learning algorithms. Such data can be used to predict purchasing habits, market trends, and other useful insights. Later, these will be helpful in decision making, streamlining inventory management, optimizing marketing platforms, and so on.
Upgrading Automation: Machine learning algorithms can evaluate the existing manufacturing models and discover their shortcomings and pain points to improve the automation of such processes. Combining automation techniques with machine learning can lead to automation processes that are constantly improving.
Cyber Security: ML algorithms can predict the nature of future cyberattacks by studying past attack data and finding the vulnerabilities in the system. Also, incorporating ML in spam filters can improve the accuracy of the filter as the efficiency of the algorithm increases with each filtering.
Conclusion
Looking through the types and applications of AI and machine learning, we can see that they overlap a lot in terms of their working and their functions. AI & ML are not that different from each other, as ML is essentially a part of AI. It concludes that all ML is AI, but not all AI is ML.
Artificial intelligence along with machine learning can power up every industry and enterprise by automating tasks, generating valuable insights, and efficiently managing resources. Eventually, as artificial intelligence evolves, powered with ML, it’ll be able to do tasks better than humans. Soon, most of the repetitive, high-volume, and rule-based business processes will be taken over by AI.

In a world with virtual assistants and smart robots, nearly every man is familiar with the terms artificial intelligence and machine learning. We know that AI & ML are the hearts behind every savvy system that stuns us, but most of us still have no idea what they are and what actually differentiates them from each other.
The terms artificial intelligence and machine learning are often used interchangeably today since people think that they’re basically the same when they aren’t. The poor definitions are what arises the confusion between AI & ML.
While artificial intelligence is a vast area of topics, machine learning is only a small part of it. AI is about building a computer system that can mimic human intelligence so that pre-programming is not required and algorithms that can work with their own intellect are used in such systems.
The tasks that come under “artificial intelligence” include problem-solving, learning, and planning which are accomplished through feeding data to the algorithms so that they can replicate such behaviors of the human brain.
With the surge in the amount of information being generated and available for analysis, researchers found that this data can be used to teach machines to perform tasks and then give them access to the internet and all the information it holds to learn themselves.
This gave birth to machine learning, which comprises the learning part of AI unlike replicating the actual human behaviors. It is a subset of AI that fosters a computer system to take decisions and make predictions based on the past data collected and just like AI systems, pre-programming isn’t required.
Before ML algorithms, AI programs were only able to perform and automate low-level tasks. With the advent of ML, AI could execute tasks beyond what they were programmed to do and the systems started evolving with each iteration.
ML algorithms can analyze large chunks of data quickly unlike the human brain, making pattern detection and prediction much easier. Structured and semi-structured data are fed into the machine learning algorithms so that they can recognize patterns in the occurrence of results and produce accurate predictions analyzing those patterns. ML algorithms change over time and get better at tasks as they interpret more and more data.
While AI covers a large set of domains and can handle multiple, complex tasks, machine learning algorithms are restricted to specific domains on which they’re trained. For instance, if we generate and train an algorithm using existing data to differentiate plants from animals based on their features, it’ll be restricted to doing that single task and will be unresponsive to other data.

Types of AI
Artificial intelligence is classified into three essential groups based on its capabilities; narrow, general, and strong AI.
Narrow AI enables the computer system to perform a specific task and cannot perform beyond its scope. Virtual assistants which operate with a limited pre-defined range of functions are an instance of narrow AI. Speech recognition, image recognition, and self-driving cars are some other examples.
General AI can perform intellectual tasks efficiently just like a human. It aims at making a system as smart as a human and currently such a system doesn’t exist which can perform tasks as perfectly as a human. General AI is still under development since researchers aren’t still able to develop a system even as intelligent as a six-year-old.
Super AI comprises systems that can beat human intelligence and can perform tasks better than any human. This can be seen as a product of general AI. The ability to think, reason, solve puzzles, learn, make decisions are all characteristics of super AI.
Use Cases of AI
Personal assistants: Assigning basic tasks and simple daily chores to virtual assistants have already become a part of our daily routine. The virtual assistant market is expanding quickly and tech giants are competing to take over the market which is going to grow in demand in the future. Even though there are concerns about user privacy, people still don’t seem to hate their Siri, Alexa, or Google Home.
Digital marketing: Though this is a new scene for AI, companies have already started deploying AI bots to optimize their marketing activities. AI tools are used for content generation in written, audio, or video format, and leaving such tasks to AI is turning out to save time and resources for companies.
Customer Service: For customers wanting instant answers to their questions, AI chatbots are proving to be very effective. Powered with voice recognition, and personalized recommendations, these chatbots are pre-programmed to answer any basic frequently asked queries and guide the customer through the website in case of an issue.
Types of ML
There are three types of machine learning, based on the nature of data available for training the algorithms; supervised, unsupervised, and reinforcement learning.
In supervised learning, the ML algorithm is trained using accurately labeled data from the training dataset. This training dataset should be similar to the dataset that we’ll input later to solve problems. The algorithm will find relationships between the parameters in the training data and deploy these findings on the final dataset.
Unsupervised learning works on raw, unlabeled data, enabling working on large datasets, without the need of them being machine-readable. Unlike supervised learning, instead of finding relationships in the training data, unsupervised learning algorithms discover the hidden structures and patterns and uncover insights from them.
Reinforcement learning is very similar to the learning process of a human. Here, the algorithm is introduced into a work environment instead of feeding already existing data. It is a feedback-based, trial and error method, where the algorithm is reinforced for every right output and discouraged for every wrong output.
Use Cases of ML
User behavior analysis: The large amount of data generated by businesses over the years can be put to good use using machine learning algorithms. Such data can be used to predict purchasing habits, market trends, and other useful insights. Later, these will be helpful in decision making, streamlining inventory management, optimizing marketing platforms, and so on.
Upgrading Automation: Machine learning algorithms can evaluate the existing manufacturing models and discover their shortcomings and pain points to improve the automation of such processes. Combining automation techniques with machine learning can lead to automation processes that are constantly improving.
Cyber Security: ML algorithms can predict the nature of future cyberattacks by studying past attack data and finding the vulnerabilities in the system. Also, incorporating ML in spam filters can improve the accuracy of the filter as the efficiency of the algorithm increases with each filtering.
Conclusion
Looking through the types and applications of AI and machine learning, we can see that they overlap a lot in terms of their working and their functions. AI & ML are not that different from each other, as ML is essentially a part of AI. It concludes that all ML is AI, but not all AI is ML.
Artificial intelligence along with machine learning can power up every industry and enterprise by automating tasks, generating valuable insights, and efficiently managing resources. Eventually, as artificial intelligence evolves, powered with ML, it’ll be able to do tasks better than humans. Soon, most of the repetitive, high-volume, and rule-based business processes will be taken over by AI.

In a world with virtual assistants and smart robots, nearly every man is familiar with the terms artificial intelligence and machine learning. We know that AI & ML are the hearts behind every savvy system that stuns us, but most of us still have no idea what they are and what actually differentiates them from each other.
The terms artificial intelligence and machine learning are often used interchangeably today since people think that they’re basically the same when they aren’t. The poor definitions are what arises the confusion between AI & ML.
While artificial intelligence is a vast area of topics, machine learning is only a small part of it. AI is about building a computer system that can mimic human intelligence so that pre-programming is not required and algorithms that can work with their own intellect are used in such systems.
The tasks that come under “artificial intelligence” include problem-solving, learning, and planning which are accomplished through feeding data to the algorithms so that they can replicate such behaviors of the human brain.
With the surge in the amount of information being generated and available for analysis, researchers found that this data can be used to teach machines to perform tasks and then give them access to the internet and all the information it holds to learn themselves.
This gave birth to machine learning, which comprises the learning part of AI unlike replicating the actual human behaviors. It is a subset of AI that fosters a computer system to take decisions and make predictions based on the past data collected and just like AI systems, pre-programming isn’t required.
Before ML algorithms, AI programs were only able to perform and automate low-level tasks. With the advent of ML, AI could execute tasks beyond what they were programmed to do and the systems started evolving with each iteration.
ML algorithms can analyze large chunks of data quickly unlike the human brain, making pattern detection and prediction much easier. Structured and semi-structured data are fed into the machine learning algorithms so that they can recognize patterns in the occurrence of results and produce accurate predictions analyzing those patterns. ML algorithms change over time and get better at tasks as they interpret more and more data.
While AI covers a large set of domains and can handle multiple, complex tasks, machine learning algorithms are restricted to specific domains on which they’re trained. For instance, if we generate and train an algorithm using existing data to differentiate plants from animals based on their features, it’ll be restricted to doing that single task and will be unresponsive to other data.

Types of AI
Artificial intelligence is classified into three essential groups based on its capabilities; narrow, general, and strong AI.
Narrow AI enables the computer system to perform a specific task and cannot perform beyond its scope. Virtual assistants which operate with a limited pre-defined range of functions are an instance of narrow AI. Speech recognition, image recognition, and self-driving cars are some other examples.
General AI can perform intellectual tasks efficiently just like a human. It aims at making a system as smart as a human and currently such a system doesn’t exist which can perform tasks as perfectly as a human. General AI is still under development since researchers aren’t still able to develop a system even as intelligent as a six-year-old.
Super AI comprises systems that can beat human intelligence and can perform tasks better than any human. This can be seen as a product of general AI. The ability to think, reason, solve puzzles, learn, make decisions are all characteristics of super AI.
Use Cases of AI
Personal assistants: Assigning basic tasks and simple daily chores to virtual assistants have already become a part of our daily routine. The virtual assistant market is expanding quickly and tech giants are competing to take over the market which is going to grow in demand in the future. Even though there are concerns about user privacy, people still don’t seem to hate their Siri, Alexa, or Google Home.
Digital marketing: Though this is a new scene for AI, companies have already started deploying AI bots to optimize their marketing activities. AI tools are used for content generation in written, audio, or video format, and leaving such tasks to AI is turning out to save time and resources for companies.
Customer Service: For customers wanting instant answers to their questions, AI chatbots are proving to be very effective. Powered with voice recognition, and personalized recommendations, these chatbots are pre-programmed to answer any basic frequently asked queries and guide the customer through the website in case of an issue.
Types of ML
There are three types of machine learning, based on the nature of data available for training the algorithms; supervised, unsupervised, and reinforcement learning.
In supervised learning, the ML algorithm is trained using accurately labeled data from the training dataset. This training dataset should be similar to the dataset that we’ll input later to solve problems. The algorithm will find relationships between the parameters in the training data and deploy these findings on the final dataset.
Unsupervised learning works on raw, unlabeled data, enabling working on large datasets, without the need of them being machine-readable. Unlike supervised learning, instead of finding relationships in the training data, unsupervised learning algorithms discover the hidden structures and patterns and uncover insights from them.
Reinforcement learning is very similar to the learning process of a human. Here, the algorithm is introduced into a work environment instead of feeding already existing data. It is a feedback-based, trial and error method, where the algorithm is reinforced for every right output and discouraged for every wrong output.
Use Cases of ML
User behavior analysis: The large amount of data generated by businesses over the years can be put to good use using machine learning algorithms. Such data can be used to predict purchasing habits, market trends, and other useful insights. Later, these will be helpful in decision making, streamlining inventory management, optimizing marketing platforms, and so on.
Upgrading Automation: Machine learning algorithms can evaluate the existing manufacturing models and discover their shortcomings and pain points to improve the automation of such processes. Combining automation techniques with machine learning can lead to automation processes that are constantly improving.
Cyber Security: ML algorithms can predict the nature of future cyberattacks by studying past attack data and finding the vulnerabilities in the system. Also, incorporating ML in spam filters can improve the accuracy of the filter as the efficiency of the algorithm increases with each filtering.
Conclusion
Looking through the types and applications of AI and machine learning, we can see that they overlap a lot in terms of their working and their functions. AI & ML are not that different from each other, as ML is essentially a part of AI. It concludes that all ML is AI, but not all AI is ML.
Artificial intelligence along with machine learning can power up every industry and enterprise by automating tasks, generating valuable insights, and efficiently managing resources. Eventually, as artificial intelligence evolves, powered with ML, it’ll be able to do tasks better than humans. Soon, most of the repetitive, high-volume, and rule-based business processes will be taken over by AI.

In a world with virtual assistants and smart robots, nearly every man is familiar with the terms artificial intelligence and machine learning. We know that AI & ML are the hearts behind every savvy system that stuns us, but most of us still have no idea what they are and what actually differentiates them from each other.
The terms artificial intelligence and machine learning are often used interchangeably today since people think that they’re basically the same when they aren’t. The poor definitions are what arises the confusion between AI & ML.
While artificial intelligence is a vast area of topics, machine learning is only a small part of it. AI is about building a computer system that can mimic human intelligence so that pre-programming is not required and algorithms that can work with their own intellect are used in such systems.
The tasks that come under “artificial intelligence” include problem-solving, learning, and planning which are accomplished through feeding data to the algorithms so that they can replicate such behaviors of the human brain.
With the surge in the amount of information being generated and available for analysis, researchers found that this data can be used to teach machines to perform tasks and then give them access to the internet and all the information it holds to learn themselves.
This gave birth to machine learning, which comprises the learning part of AI unlike replicating the actual human behaviors. It is a subset of AI that fosters a computer system to take decisions and make predictions based on the past data collected and just like AI systems, pre-programming isn’t required.
Before ML algorithms, AI programs were only able to perform and automate low-level tasks. With the advent of ML, AI could execute tasks beyond what they were programmed to do and the systems started evolving with each iteration.
ML algorithms can analyze large chunks of data quickly unlike the human brain, making pattern detection and prediction much easier. Structured and semi-structured data are fed into the machine learning algorithms so that they can recognize patterns in the occurrence of results and produce accurate predictions analyzing those patterns. ML algorithms change over time and get better at tasks as they interpret more and more data.
While AI covers a large set of domains and can handle multiple, complex tasks, machine learning algorithms are restricted to specific domains on which they’re trained. For instance, if we generate and train an algorithm using existing data to differentiate plants from animals based on their features, it’ll be restricted to doing that single task and will be unresponsive to other data.

Types of AI
Artificial intelligence is classified into three essential groups based on its capabilities; narrow, general, and strong AI.
Narrow AI enables the computer system to perform a specific task and cannot perform beyond its scope. Virtual assistants which operate with a limited pre-defined range of functions are an instance of narrow AI. Speech recognition, image recognition, and self-driving cars are some other examples.
General AI can perform intellectual tasks efficiently just like a human. It aims at making a system as smart as a human and currently such a system doesn’t exist which can perform tasks as perfectly as a human. General AI is still under development since researchers aren’t still able to develop a system even as intelligent as a six-year-old.
Super AI comprises systems that can beat human intelligence and can perform tasks better than any human. This can be seen as a product of general AI. The ability to think, reason, solve puzzles, learn, make decisions are all characteristics of super AI.
Use Cases of AI
Personal assistants: Assigning basic tasks and simple daily chores to virtual assistants have already become a part of our daily routine. The virtual assistant market is expanding quickly and tech giants are competing to take over the market which is going to grow in demand in the future. Even though there are concerns about user privacy, people still don’t seem to hate their Siri, Alexa, or Google Home.
Digital marketing: Though this is a new scene for AI, companies have already started deploying AI bots to optimize their marketing activities. AI tools are used for content generation in written, audio, or video format, and leaving such tasks to AI is turning out to save time and resources for companies.
Customer Service: For customers wanting instant answers to their questions, AI chatbots are proving to be very effective. Powered with voice recognition, and personalized recommendations, these chatbots are pre-programmed to answer any basic frequently asked queries and guide the customer through the website in case of an issue.
Types of ML
There are three types of machine learning, based on the nature of data available for training the algorithms; supervised, unsupervised, and reinforcement learning.
In supervised learning, the ML algorithm is trained using accurately labeled data from the training dataset. This training dataset should be similar to the dataset that we’ll input later to solve problems. The algorithm will find relationships between the parameters in the training data and deploy these findings on the final dataset.
Unsupervised learning works on raw, unlabeled data, enabling working on large datasets, without the need of them being machine-readable. Unlike supervised learning, instead of finding relationships in the training data, unsupervised learning algorithms discover the hidden structures and patterns and uncover insights from them.
Reinforcement learning is very similar to the learning process of a human. Here, the algorithm is introduced into a work environment instead of feeding already existing data. It is a feedback-based, trial and error method, where the algorithm is reinforced for every right output and discouraged for every wrong output.
Use Cases of ML
User behavior analysis: The large amount of data generated by businesses over the years can be put to good use using machine learning algorithms. Such data can be used to predict purchasing habits, market trends, and other useful insights. Later, these will be helpful in decision making, streamlining inventory management, optimizing marketing platforms, and so on.
Upgrading Automation: Machine learning algorithms can evaluate the existing manufacturing models and discover their shortcomings and pain points to improve the automation of such processes. Combining automation techniques with machine learning can lead to automation processes that are constantly improving.
Cyber Security: ML algorithms can predict the nature of future cyberattacks by studying past attack data and finding the vulnerabilities in the system. Also, incorporating ML in spam filters can improve the accuracy of the filter as the efficiency of the algorithm increases with each filtering.
Conclusion
Looking through the types and applications of AI and machine learning, we can see that they overlap a lot in terms of their working and their functions. AI & ML are not that different from each other, as ML is essentially a part of AI. It concludes that all ML is AI, but not all AI is ML.
Artificial intelligence along with machine learning can power up every industry and enterprise by automating tasks, generating valuable insights, and efficiently managing resources. Eventually, as artificial intelligence evolves, powered with ML, it’ll be able to do tasks better than humans. Soon, most of the repetitive, high-volume, and rule-based business processes will be taken over by AI.

Recent Posts
Transform your vision into reality with Custom Software Development
Get Started
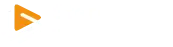
Office Address:
743A, Gera’s Imperium Rise,Hinjewadi Phase II, Rajiv Gandhi Infotech Park, Near Wipro Circle, Pune- 411057, Maharashtra, India
Transform your vision into reality with Custom Software Development
Get Started
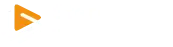
Office Address:
743A, Gera’s Imperium Rise,Hinjewadi Phase II, Rajiv Gandhi Infotech Park, Near Wipro Circle, Pune- 411057, Maharashtra, India
Transform your vision into reality with Custom Software Development
Get Started
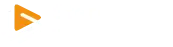
Office Address:
743A, Gera’s Imperium Rise,Hinjewadi Phase II, Rajiv Gandhi Infotech Park, Near Wipro Circle, Pune- 411057, Maharashtra, India
Transform your vision into reality with Custom Software Development
Get Started
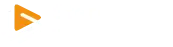
Office Address:
743A, Gera’s Imperium Rise,Hinjewadi Phase II, Rajiv Gandhi Infotech Park, Near Wipro Circle, Pune- 411057, Maharashtra, India
Transform your vision into reality with Custom Software Development
Get Started
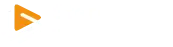
Office Address:
743A, Gera’s Imperium Rise,Hinjewadi Phase II, Rajiv Gandhi Infotech Park, Near Wipro Circle, Pune- 411057, Maharashtra, India
Transform your vision into reality with Custom Software Development
Get Started
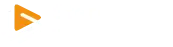
Office Address:
743A, Gera’s Imperium Rise,Hinjewadi Phase II, Rajiv Gandhi Infotech Park, Near Wipro Circle, Pune- 411057, Maharashtra, India